Venture Building in the field of AI
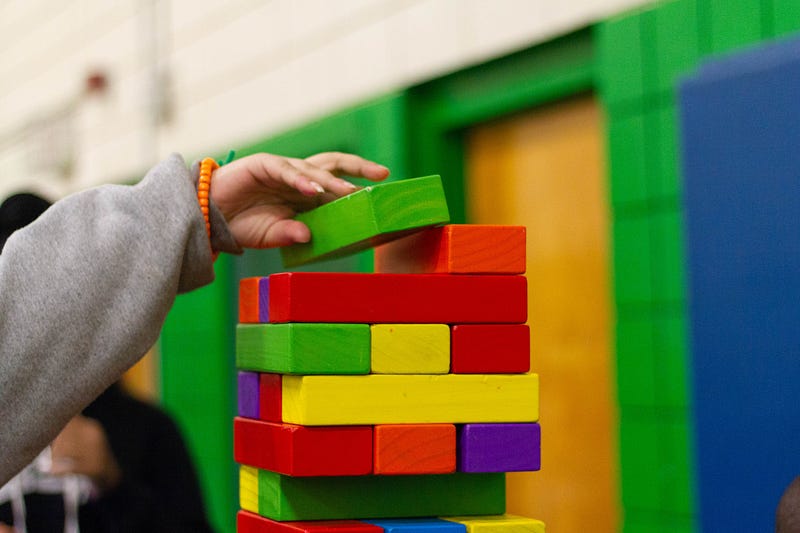
Venture building in the field of AI has seen a lot of traction. Many venture funds exist and they also keep on announcing newer funds dedicated to finding innovations in AI.
If you are a founder and want to know the phase such venture development is in, then this is the article for you.
The basic tenant
AI venture development is all about finding the “unique data set that has a predictive value”.
Most of the funding so far has been in the venture where the entrepreneurs were able to collect a unique data set, built models around that, and then talked to a company or two and found interest.
AI Venture Categories
Now let’s try to classify AI ventures in different categories.
We believe that in terms of chronological order and risk appetite, four broader categories of AI Supplemented, AI Centric, AI-First, AI-Enablement applications, emerge. Let’s discuss those.
AI Supplemented Applications
Initial traction in AI came from Deep Learning. And the ideal utility for that technique was “search”. So, a lot of innovation came through classifiers and recommendation systems.
And all such implementations were done in the consumer domain.
At this stage, the ecosystem was warming up to the possibilities of the AI, so risk-taking was at the minimum.
With success came a wave of series of ventures where AI was supplemented to add intelligence to the applications. For example, many popular CRMs and marketing automation tools started using AI for intelligence.
In this category, applications worked without AI. AI gave them the option of making their systems “smart”.
AI-Centric Applications
Once the AI supplemented applications got some traction, the risk appetite increased and investors and entrepreneurs moved towards building AI Centric Applications.
Here the idea was to build a system where AI is the central component. If AI doesn’t work, the system doesn’t work.
The ideal candidate for such exploration was perception.
With advances in computer vision and NLP, autonomy became the next frontier to be explored. Also, AI made autonomy cheap.
So, self-driving cars, robots doing inventory, drones supplying blood, and so on, became the obsession. Still very relevant in 2020.
AI-First Applications
Here innovators are challenging the norms and using AI to work with complex systems.
You can think in terms of Google’s “Deep Mind”. Google used it to reduce its data center energy consumption costs. The system went through thousands of different variables and reduced energy consumption by a whopping 40%.
With AI-First applications, innovators will go deep into complex problems. It will be related to finding solutions for agriculture, logistics, distribution, retail, and so.
AI-Enablement Applications
So, all the above categories are examples of using AI for a niche or an industry vertical. But there are other tools that enable engineers to use AI modeling easily. Those are in effect industry-agnostic.
You can think of such tools in terms of building shovels and tools for the actual work. Take the case of Databricks. It is one of the tools used for processing and transforming massive quantities of data and exploring the data through machine learning models.
Here the major competition comes from AWS, Azure, and Google. All the major cloud providers are giving free tools to enable customers to bring their workloads online. This tactic helps cloud vendors to lock in customers early and then charge them later for more computational power.