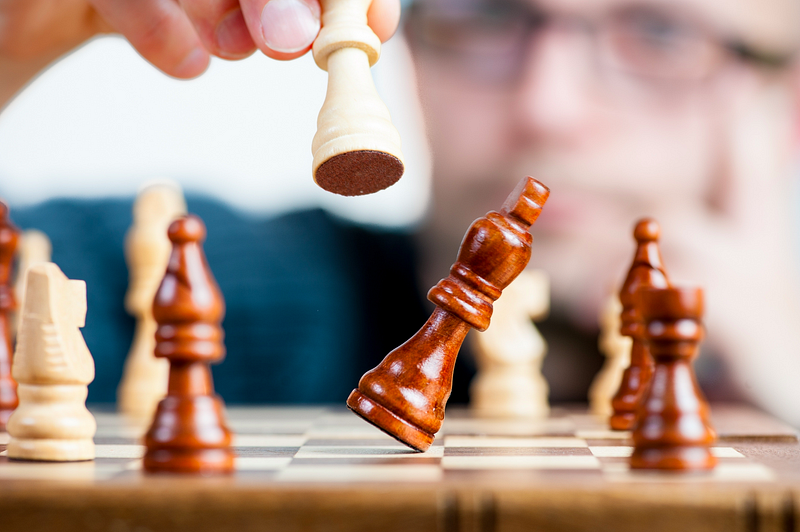
Artificial intelligence will either take over or at least augment the decision-making process from executives to front line workers. With much-anticipated disruption projected, organizations are working with AI initiatives and spending on acquiring talent and data. Yet, as per surveys, the progress is slow and there are many examples for misfires.
Mostly the failures are attributed to high-level organizational design and rigidity. Organizations are always looking to transition from rigid, siloed, risk-averse mindset to agile, experimental, and data-driven cultures. But the agility goals are broad and don’t add much to the tactical preparation for any company to execute AI well.
So, let’s look at some of the AI production and consumption strategies of organizations, that have scaled well with AI and led its adoption, have followed.
AI Production Tactics
From the AI production strategy, the first question that impacts any leader is the debate of where to house the project. Leaders need to navigate the organizations and determine what organizational model and under what leader (C-Level) should it be hosted.
So from a production point of view, there are two obvious strategies of either centralization or decentralization.
Let’s talk about those.
Centralization presents the option of building central functional capabilities and then distributing tools to the individual business units for operations.
Andrew NG talks about such initiatives and uses the lack of quality resources as the basis of his argument.
As per Andrew, there is a shortage of AI technical resources, also the field is evolving, and the supply isn’t matching the demand. He compares this lack of quality resources to the mobile developers’ shortage 10 years back. At that time, the organizations built the Central Repository of skills and provided skills and resources as needed by each division or business function.
Decentralization is the other option where AI production and talent development is run by business divisions as per the use cases they encounter.
With this approach, the issues could stem not only from the talent perspective but also from misalignment of initiatives, lack of directions, and prioritization of right use cases.
Interestingly, HBR has found examples of success with each of the above strategies with companies competing in the same domain. AI initiatives of such companies witnessed scale and growth.
Let’s look at some of the components that drive a push towards the centralization or distribution of AI Production.
AI Maturity
From a company’s perspective, the most important factor to consider is the stage of AI maturity the company is in. This not only includes talent like analytical engineers, data scientists, data engineers and so on, but also the strategy, processes, and use cases discovered so far.
For a company that’s early in this process, it makes a lot more sense for the C-level executives to sit with data scientists and machine learning engineers, and to come up with strategies and use cases that can help them prove the model. In such cases, centralization gives a company the ability to get the direction right and to scale.
Business Model
AI is meant to augment existing capabilities of an organization and give the companies a competitive edge over the competition to do well. The complexity of business processes can be a major hurdle to change and the adoption of new tools. In such cases, where there are many business intricacies, the case of building AI centrally makes a lot more sense.
On the contrary, if the business model and processes are simple, and the company has access to capabilities across geographies or divisions, it makes sense to decentralize AI initiative. This way there is a chance of finding “winners” from a broad range of use cases as companies get access to different creative ideas.
Talent Access
Skill and talent access should be a central decision making a factor. Considering the state the industry is in, where the demand continues to outstrip the supply, the best possible way would be to start building a centralized AI team.
Organization Design
One of the major challenges could come in the form of the company current’s organizational structure. Since AI adoption involves a multi-disciplinary team working together, the work patterns might involve a matrix structure and that has inherent frictional issues.
If the organization is functionally structured, the change and getting into a new collaborative way of working become challenging.
Now, let’s look at some of the ways to make AI consumption a success
Skill Development
For AI initiatives to succeed, the leadership and the AI team, both need to stay abreast of the latest AI trends and techniques.
For leadership, the macro-level understanding and also the know-how of usable and successful business cases are a must.
For data scientists, developers, and analytical engineers, much focus should on sharpening the hard skills of AI and keep track of all the latest algorithmic innovations and successful deployments.
Workers also need to be made aware of the changes that might pop up in their work domain. In most of the cases, where AI ends up augmenting their capabilities, a new set of training and skill development will be needed to work with AI tools.
For teams like marketing, finance, and HR, higher-level training is needed to stay abreast of all the changes that can help improve their productivity.
Change Management
Change management hinges upon the leadership willingness to stick and commit to the proposed new way of doing. AI implementation would require the same.
As per HBR, most of the initiatives are taking at least 2–3 years to complete. So, as leaders, you need to encourage all the experimentation and encourage risk-taking for an extended time period.
Input for better iterations
For every business unit, planning to deploy AI-based tools, should also have the ability to give feedback about the efficacy of interfaces and the results. The incorporation of such feedback is gold and will only help the leaders improve their models, use cases, and commitment to AI.
Also, any feedback, where it yields improvements, should be welcomed and highlighted at the leadership level.
Alignment of incentives
People move in directions where they are awarded or incentivized.
In case of initiatives that encapsulate multiple business divisions and involve multidisciplinary teams, the role of a balanced scorecard and shared responsibility, increases. The best firms should not only empower their employees but also incentivize them for the right behavior and eventual business outcomes.
Communication
Remember your employees could be suspicious of the role AI is going to play. With AI, at times, it’s complete reengineering of work and processes. So, communicating with employees, showing them use cases where their productivity increases, and finally sharing the business impact, is crucial.
Summing Up
AI will definitely change the way we interact with the world and the way we make decisions. Companies will have to change and meet the challenges of AI production and consumption. And those that will excel at such capabilities will find them competing at a higher level, with their processes and decision-making abilities being augmented with intelligent machines.